Tell me more about homomorphic encryption
Asked on 08/01/2024
1 search
Homomorphic encryption is a form of encryption that allows computations to be performed on encrypted data without needing to decrypt it first. This ensures that the data remains secure and private even while being processed.
At WWDC 2024, homomorphic encryption was mentioned in the context of privacy enhancements for Live Caller ID. Specifically, it was highlighted in the session titled "What’s new in privacy." The implementation of private information retrieval in Live Caller ID uses homomorphic encryption to enable a server to make use of an encrypted value without decrypting it. The server computes on the incoming ciphertext, evaluates for a match, and then returns the transformed ciphertext back to the requesting device, where the results are displayed. This allows for the implementation of Live Caller ID without revealing sensitive information such as an incoming phone number.
For more details, you can refer to the session What’s new in privacy (21:32).
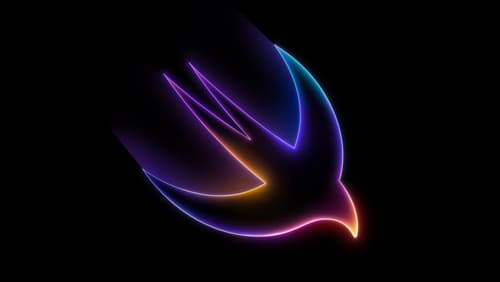
Platforms State of the Union
Discover the newest advancements on Apple platforms.
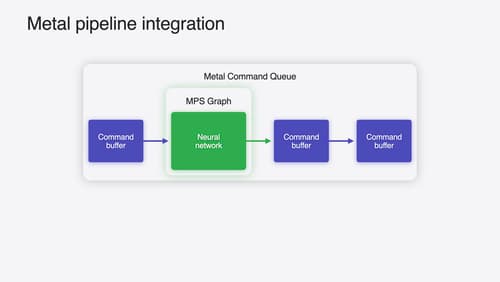
Accelerate machine learning with Metal
Learn how to accelerate your machine learning transformer models with new features in Metal Performance Shaders Graph. We’ll also cover how to improve your model’s compute bandwidth and quality, and visualize it in the all new MPSGraph viewer.
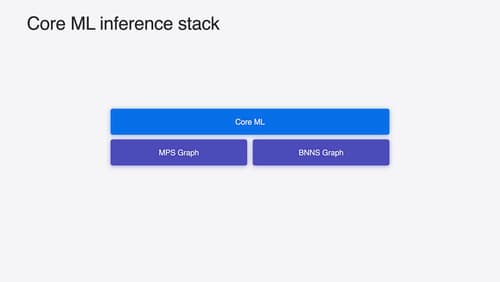
Deploy machine learning and AI models on-device with Core ML
Learn new ways to optimize speed and memory performance when you convert and run machine learning and AI models through Core ML. We’ll cover new options for model representations, performance insights, execution, and model stitching which can be used together to create compelling and private on-device experiences.