CoreML에서 Model with state인 내용을 자세히 설명해줘 한글로
Asked on 08/12/2024
1 search
CoreML에서 "Model with state"에 대한 설명은 다음과 같습니다.
CoreML은 이제 상태를 가진 모델(stateful model)을 지원합니다. 상태를 가진 모델은 입력과 출력을 처리할 때 이전의 입력 데이터를 기억하고 활용할 수 있는 모델을 의미합니다. 예를 들어, 누적기(accumulator) 모델은 모든 과거 입력의 합계를 추적합니다. 상태는 초기화된 후, 모델이 상태에서 값을 읽고 입력과 함께 출력을 생성하며, 결과를 다시 상태에 기록합니다. 이렇게 하면 다음 입력이 들어올 때 상태를 업데이트하여 사용할 수 있습니다.
이전에는 상태를 관리하기 위해 추가적인 입력과 출력을 명시적으로 정의해야 했지만, CoreML의 새로운 기능을 통해 상태 텐서를 자동으로 업데이트할 수 있게 되었습니다. 이는 성능을 향상시키며, 특히 상태가 큰 경우에 효율적입니다.
PyTorch에서 상태 텐서를 정의할 때는 register_buffer
API를 사용하여 상태를 지정하고, CoreML로 변환할 때는 새로 도입된 CT state type
을 사용하여 상태를 지정합니다. 이렇게 변환된 CoreML 모델은 상태를 가진 모델로, 입력과 출력 외에 상태를 관리할 수 있습니다.
이와 관련된 자세한 내용은 Bring your machine learning and AI models to Apple silicon 세션에서 확인할 수 있습니다.
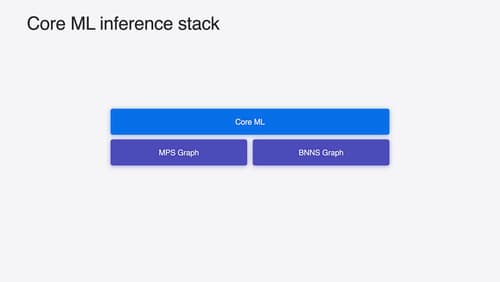
Deploy machine learning and AI models on-device with Core ML
Learn new ways to optimize speed and memory performance when you convert and run machine learning and AI models through Core ML. We’ll cover new options for model representations, performance insights, execution, and model stitching which can be used together to create compelling and private on-device experiences.
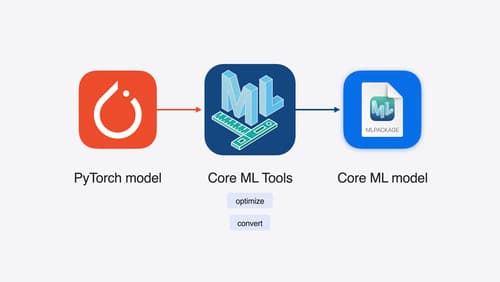
Bring your machine learning and AI models to Apple silicon
Learn how to optimize your machine learning and AI models to leverage the power of Apple silicon. Review model conversion workflows to prepare your models for on-device deployment. Understand model compression techniques that are compatible with Apple silicon, and at what stages in your model deployment workflow you can apply them. We’ll also explore the tradeoffs between storage size, latency, power usage and accuracy.
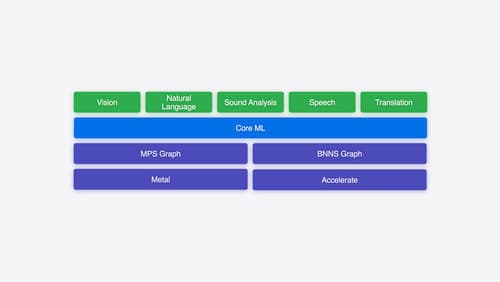
Explore machine learning on Apple platforms
Get started with an overview of machine learning frameworks on Apple platforms. Whether you’re implementing your first ML model, or an ML expert, we’ll offer guidance to help you select the right framework for your app’s needs.