Transformer optimization에 대해 자세히 설명해줘, 한글로!
Asked on 08/12/2024
1 search
트랜스포머 최적화에 대해 설명드리겠습니다. 트랜스포머 모델은 주로 언어 모델에서 사용되며, 텍스트를 번역, 예측 및 생성하는 데 활용됩니다. 트랜스포머 모델의 핵심은 주의 메커니즘(Attention Mechanism)입니다. 이 메커니즘은 입력된 토큰화된 텍스트를 쿼리, 키, 값 텐서로 나누고, 이 텐서들을 행렬 곱셈, 스케일링, 소프트맥스 연산을 통해 조작합니다.
Apple의 GPU에서는 이러한 일련의 연산을 단일 커널 호출로 융합하여, 여러 작은 연산을 GPU에 디스패치하는 오버헤드를 줄임으로써 네트워크의 전체 성능을 향상시킬 수 있습니다. 또한, Apple 기기의 통합 메모리 아키텍처 덕분에 텐서를 메인 메모리에 두고 CPU와 GPU가 메모리 복사 없이 접근할 수 있어 성능이 더욱 향상됩니다.
트랜스포머 모델의 성능을 최적화하는 방법으로는, 주의 블록 내의 연산을 최적화하는 것이 있습니다. 예를 들어, 스케일드 닷 프로덕트 어텐션(SDPA) 연산을 융합하여 효율적으로 계산할 수 있습니다. 이는 Apple 실리콘 GPU에서 특히 효과적입니다.
이와 관련된 세션으로는 Train your machine learning and AI models on Apple GPUs와 Accelerate machine learning with Metal이 있습니다. 이 세션들은 트랜스포머 모델의 최적화와 관련된 다양한 기술적 세부사항을 다루고 있습니다.
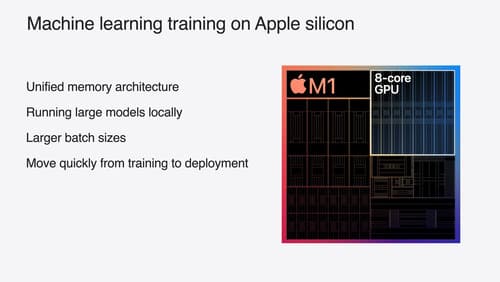
Train your machine learning and AI models on Apple GPUs
Learn how to train your models on Apple Silicon with Metal for PyTorch, JAX and TensorFlow. Take advantage of new attention operations and quantization support for improved transformer model performance on your devices.
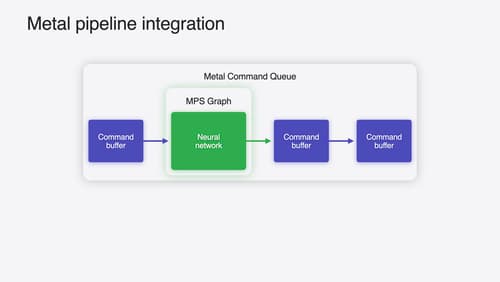
Accelerate machine learning with Metal
Learn how to accelerate your machine learning transformer models with new features in Metal Performance Shaders Graph. We’ll also cover how to improve your model’s compute bandwidth and quality, and visualize it in the all new MPSGraph viewer.
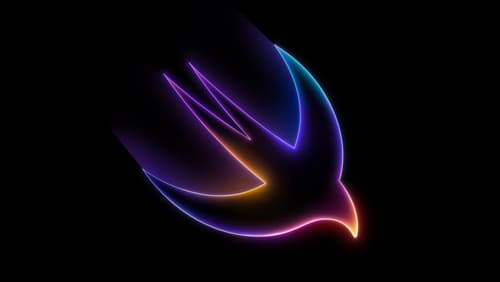
Platforms State of the Union
Discover the newest advancements on Apple platforms.