core ml channel 과 block 설명해줘
Generated on 8/20/2024
1 search
Core ML is a framework by Apple that allows developers to deploy machine learning and AI models on Apple devices. It provides tools to efficiently run models on-device, leveraging the CPU, GPU, and neural engine to maximize hardware utilization. Core ML supports features like ML tensor for simplifying model integration, multifunction models for deploying models with multiple functionalities, and performance tools for profiling and debugging models.
In the context of Core ML, a "channel" is not directly related. However, in the context of broadcasting updates to Live Activities, a channel is a mechanism to manage and send broadcast push notifications. You can create a channel for your app using the push notifications console or directly through the app server in production. This channel is used to subscribe to updates and send notifications to live activities.
For more detailed information on Core ML and its features, you can refer to the session Deploy machine learning and AI models on-device with Core ML.
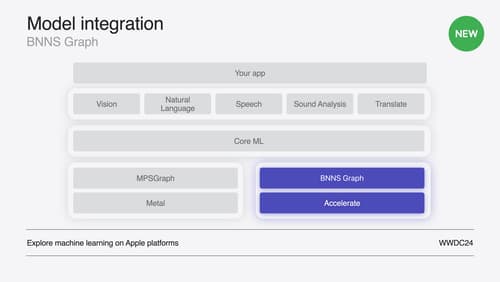
Support real-time ML inference on the CPU
Discover how you can use BNNSGraph to accelerate the execution of your machine learning model on the CPU. We will show you how to use BNNSGraph to compile and execute a machine learning model on the CPU and share how it provides real-time guarantees such as no runtime memory allocation and single-threaded running for audio or signal processing models.
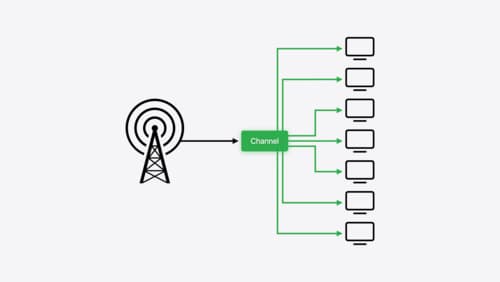
Broadcast updates to your Live Activities
With broadcast push notifications, your app can send updates to thousands of Live Activities with a single request. We’ll discover how broadcast push notifications work between an app, a server, and the Apple Push Notification service, then we’ll walk through best practices for this capability and how to implement it.
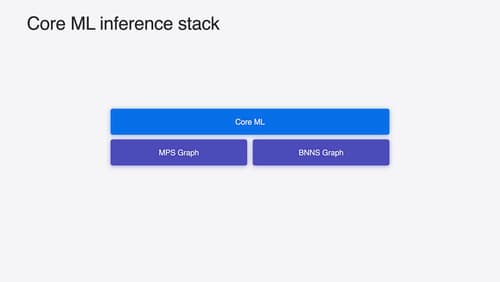
Deploy machine learning and AI models on-device with Core ML
Learn new ways to optimize speed and memory performance when you convert and run machine learning and AI models through Core ML. We’ll cover new options for model representations, performance insights, execution, and model stitching which can be used together to create compelling and private on-device experiences.
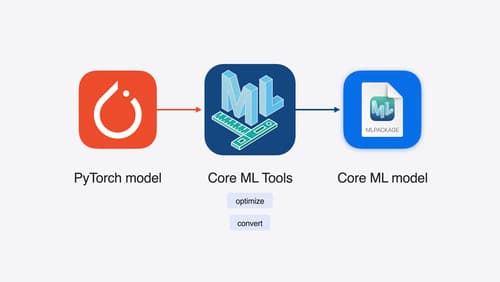
Bring your machine learning and AI models to Apple silicon
Learn how to optimize your machine learning and AI models to leverage the power of Apple silicon. Review model conversion workflows to prepare your models for on-device deployment. Understand model compression techniques that are compatible with Apple silicon, and at what stages in your model deployment workflow you can apply them. We’ll also explore the tradeoffs between storage size, latency, power usage and accuracy.
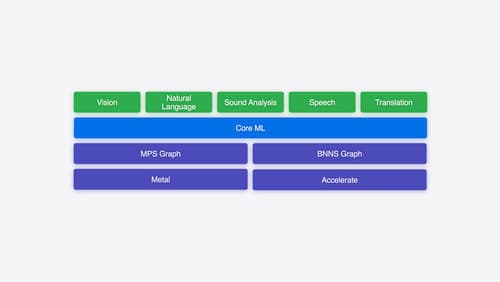
Explore machine learning on Apple platforms
Get started with an overview of machine learning frameworks on Apple platforms. Whether you’re implementing your first ML model, or an ML expert, we’ll offer guidance to help you select the right framework for your app’s needs.
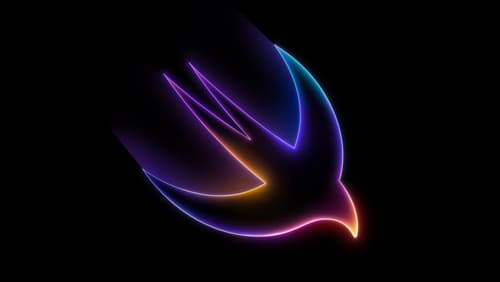
Platforms State of the Union
Discover the newest advancements on Apple platforms.