tensor flow
Generated on 8/20/2024
1 search
It seems you are interested in TensorFlow and its integration with Apple's platforms. At WWDC, Apple discussed several aspects of deploying and training machine learning models using their technologies.
Key Points from WWDC:
-
Core ML and ML Tensor:
- Core ML now supports ML Tensor, which simplifies tensor operations similar to Python numerical libraries. This is particularly useful for integrating large language models and performing operations asynchronously. Deploy machine learning and AI models on-device with Core ML.
-
Training on Apple GPUs:
- Apple provides support for training machine learning models on their GPUs using frameworks like TensorFlow, PyTorch, and JAX. The Metal backend for TensorFlow supports features like distributed training and mixed precision to enhance performance. Train your machine learning and AI models on Apple GPUs.
-
Quantization and Performance:
- Quantization techniques are used to reduce memory requirements for models, which is beneficial for deploying large models on devices. This includes support for 8-bit and 4-bit integer quantization, which helps fit larger models into device memory. Train your machine learning and AI models on Apple GPUs.
-
Metal Performance Shaders (MPS):
- MPS Graph provides low-level control over GPU synchronization and memory, which can be used to accelerate machine learning tasks. This is particularly useful for applications that already use Metal. Accelerate machine learning with Metal.
Relevant Sessions:
- Train your machine learning and AI models on Apple GPUs
- Deploy machine learning and AI models on-device with Core ML
- Accelerate machine learning with Metal
If you have more specific questions about TensorFlow or any other aspect of machine learning on Apple platforms, feel free to ask!
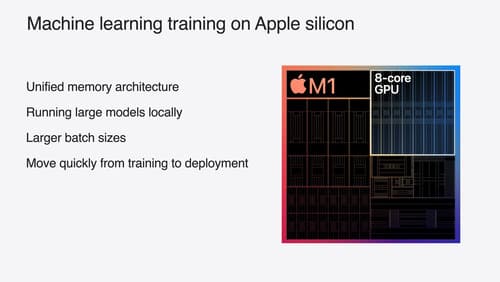
Train your machine learning and AI models on Apple GPUs
Learn how to train your models on Apple Silicon with Metal for PyTorch, JAX and TensorFlow. Take advantage of new attention operations and quantization support for improved transformer model performance on your devices.
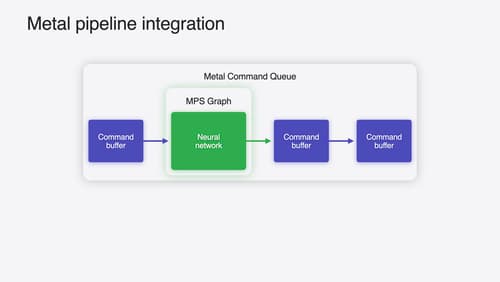
Accelerate machine learning with Metal
Learn how to accelerate your machine learning transformer models with new features in Metal Performance Shaders Graph. We’ll also cover how to improve your model’s compute bandwidth and quality, and visualize it in the all new MPSGraph viewer.
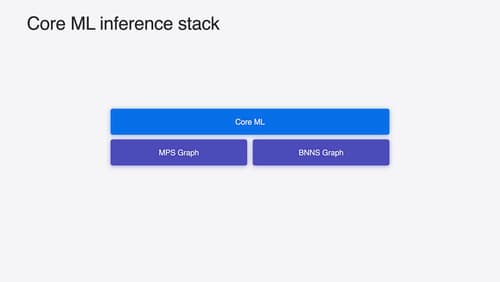
Deploy machine learning and AI models on-device with Core ML
Learn new ways to optimize speed and memory performance when you convert and run machine learning and AI models through Core ML. We’ll cover new options for model representations, performance insights, execution, and model stitching which can be used together to create compelling and private on-device experiences.