what is mixtral model
Asked on 02/17/2025
1 search
The "Mistral model" mentioned in the context refers to the Mistral 7 billion parameter model from Mistral's Hugging Face space. It is used in demonstrations at WWDC to showcase optimizations in model execution, such as quantization and stateful KV cache techniques in Core ML. These optimizations help in running the model more efficiently on Apple devices, particularly on macOS. The Mistral model is an example of how Apple is integrating advanced machine learning models into its ecosystem, allowing developers to leverage these capabilities in their applications.
For more details, you can refer to the Platforms State of the Union session.
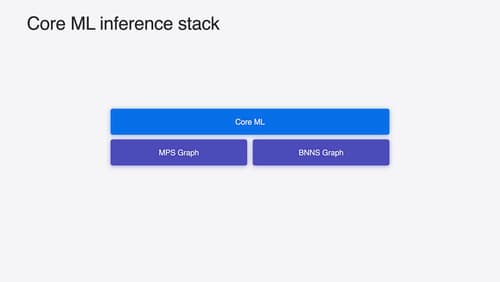
Deploy machine learning and AI models on-device with Core ML
Learn new ways to optimize speed and memory performance when you convert and run machine learning and AI models through Core ML. We’ll cover new options for model representations, performance insights, execution, and model stitching which can be used together to create compelling and private on-device experiences.
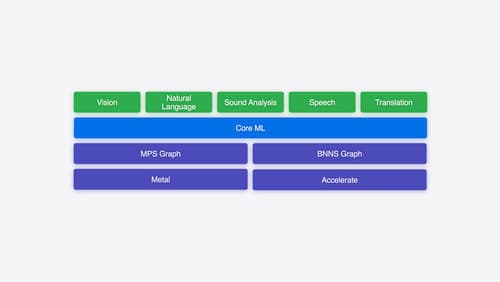
Explore machine learning on Apple platforms
Get started with an overview of machine learning frameworks on Apple platforms. Whether you’re implementing your first ML model, or an ML expert, we’ll offer guidance to help you select the right framework for your app’s needs.
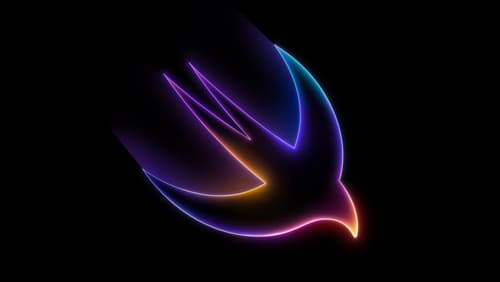
Platforms State of the Union
Discover the newest advancements on Apple platforms.