caching
Generated on 4/29/2025
1 search
Caching, particularly in the context of machine learning models, was discussed in several sessions at WWDC. A key focus was on the use of a "key-value cache" (KV cache) to improve the efficiency of transformer models, which are commonly used in large language models.
Key Points on Caching:
-
KV Cache in Transformers:
- The KV cache is used to store key and value vectors calculated at each step of the model's operation. This avoids recalculating these vectors for previous tokens, thus speeding up the decoding process.
- This technique is particularly useful for large language models to make decoding faster and more efficient.
-
Implementation in Core ML:
- In the session "Deploy machine learning and AI models on-device with Core ML," it was explained how the KV cache can be managed using Core ML states, reducing overhead and improving inference efficiency. This involves creating an empty cache to store key and value vectors, which is then updated in place.
-
Metal and Machine Learning:
- In the session "Accelerate machine learning with Metal," the use of the KV cache was also discussed. The session highlighted how to update the cache in place using operations like
sliceupdate
to optimize memory usage and computation.
- In the session "Accelerate machine learning with Metal," the use of the KV cache was also discussed. The session highlighted how to update the cache in place using operations like
Relevant Sessions:
- Deploy machine learning and AI models on-device with Core ML (Models with state)
- Bring your machine learning and AI models to Apple silicon (Transformer optimization)
- Accelerate machine learning with Metal (Transformer support)
These sessions provide insights into how caching techniques can be leveraged to enhance the performance of machine learning models on Apple devices.
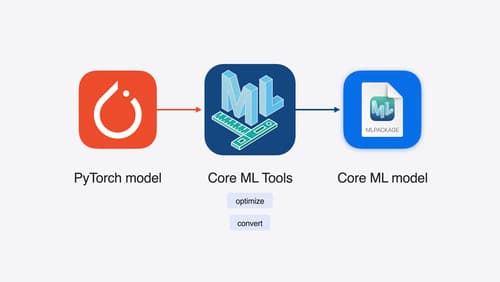
Bring your machine learning and AI models to Apple silicon
Learn how to optimize your machine learning and AI models to leverage the power of Apple silicon. Review model conversion workflows to prepare your models for on-device deployment. Understand model compression techniques that are compatible with Apple silicon, and at what stages in your model deployment workflow you can apply them. We’ll also explore the tradeoffs between storage size, latency, power usage and accuracy.
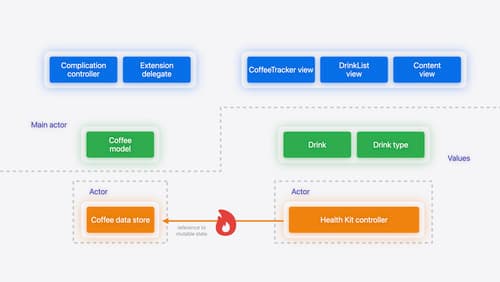
Migrate your app to Swift 6
Experience Swift 6 migration in action as we update an existing sample app. Learn how to migrate incrementally, module by module, and how the compiler helps you identify code that’s at risk of data races. Discover different techniques for ensuring clear isolation boundaries and eliminating concurrent access to shared mutable state.
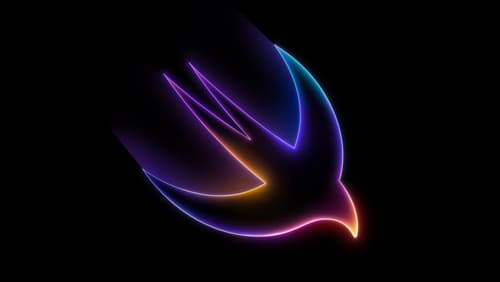
Platforms State of the Union
Discover the newest advancements on Apple platforms.
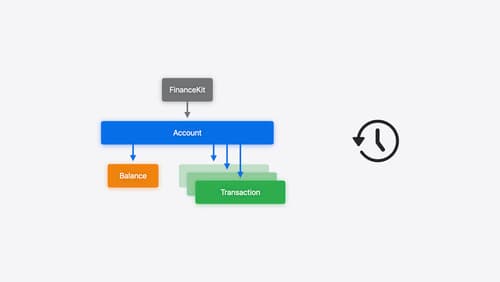
Meet FinanceKit
Learn how FinanceKit lets your financial management apps seamlessly and securely share on-device data from Apple Cash, Apple Card, and more, with user consent and control. Find out how to request one-time and ongoing access to accounts, transactions, and balances — and how to build great experiences for iOS and iPadOS.
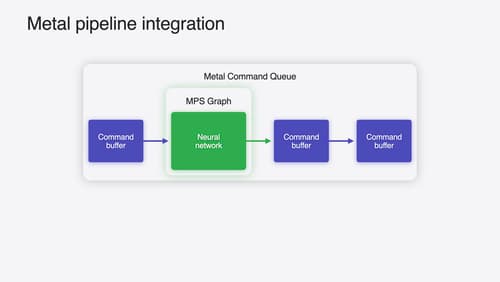
Accelerate machine learning with Metal
Learn how to accelerate your machine learning transformer models with new features in Metal Performance Shaders Graph. We’ll also cover how to improve your model’s compute bandwidth and quality, and visualize it in the all new MPSGraph viewer.
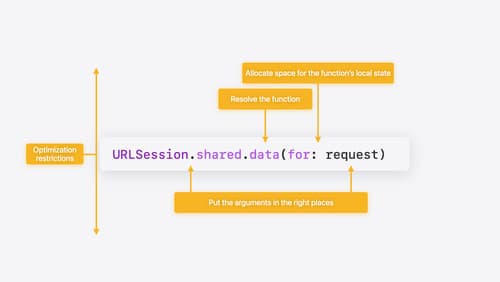
Explore Swift performance
Discover how Swift balances abstraction and performance. Learn what elements of performance to consider and how the Swift optimizer affects them. Explore the different features of Swift and how they’re implemented to further understand the tradeoffs available that can impact performance.
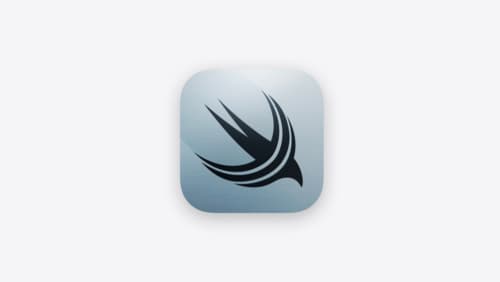
What’s new in SwiftData
SwiftData makes it easy to add persistence to your app with its expressive, declarative API. Learn about refinements to SwiftData, including compound uniqueness constraints, faster queries with #Index, queries in Xcode previews, and rich predicate expressions. Join us to explore how you can use all of these features to express richer models and improve performance in your app. To discover how to build a custom data store or use the history API in SwiftData, watch “Create a custom data store with SwiftData” and “Track model changes with SwiftData history”.
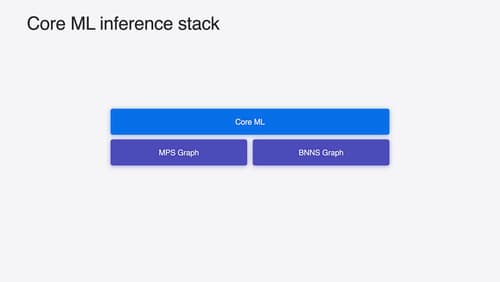
Deploy machine learning and AI models on-device with Core ML
Learn new ways to optimize speed and memory performance when you convert and run machine learning and AI models through Core ML. We’ll cover new options for model representations, performance insights, execution, and model stitching which can be used together to create compelling and private on-device experiences.
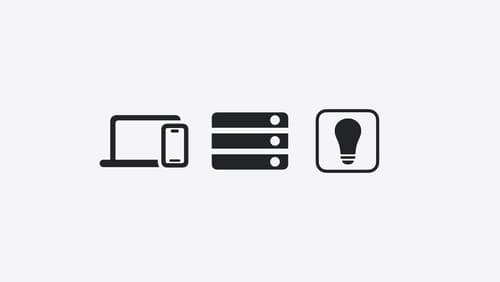
A Swift Tour: Explore Swift’s features and design
Learn the essential features and design philosophy of the Swift programming language. We’ll explore how to model data, handle errors, use protocols, write concurrent code, and more while building up a Swift package that has a library, an HTTP server, and a command line client. Whether you’re just beginning your Swift journey or have been with us from the start, this talk will help you get the most out of the language.